This is a guest post written by Robbie Jerrom, senior principal technologist for AI, AI BU Red Hat.
Jerrom writes in full as follows…
In enterprise AI deployments, model size is frequently equated with performance, under the assumption that more parameters yield better results. The more parameters, the more powerful the model – at least in theory. But in practice, especially inside enterprise environments, that assumption is giving way to something more useful: how well a model fits the task, the system and the operational realities of where it’s deployed.
In this context, the way small language models (SLMs) are being used is evolving. As industries move to integrate AI more deeply, SLMs offer a practical alternative to Large Language Models (LLMs), particularly for organisations aiming to deploy AI solutions proximal to their data sources, such as at the edge or within environments constrained by strict privacy and cost considerations.
The goal shouldn’t be to chase the biggest model. It should be to build intelligent systems that are flexible, efficient and aligned with the organisation’s architecture, applications, industry and desired outcomes.
This thinking is already reflected in how enterprises are evaluating generative AI models today, with small, specialised models playing a central role in real-world deployments. When determining trust in an enterprise model for generative AI, 79% of UK IT leaders prioritised domain-specific models over generic large language models. Proven performance in similar use cases (85%) was another key trust driver.
The AI triad
Enterprise AI can be conceptualised as a triad comprising models, applications and platforms – each integral to the system’s overall efficacy. Each of these should reinforce the other and each is likely evolving rapidly; this view is emerging as the foundational shape of intelligent IT. Models bring intelligence. Applications provide context and business function. Platforms tie it all together – delivering the infrastructure, orchestration and scale needed to make AI work effectively in practice.
Crucially, no single part of this triad can stand alone.
A powerful model without an application to embed into is just potential. An application without intelligence risks stagnation. And without the right platform to run across environments, from core to edge, neither models nor applications can perform effectively. It’s this interdependence that defines AI’s next chapter in the enterprise: not siloed innovation, but layered systems that are designed to evolve together.
This should also reframe how enterprises approach AI adoption. It’s not just a question of building or buying a model, but ensuring alignment across the triad. Can the platform support the model’s resource needs? Can the application surface the intelligence in a usable, governed way? Can all three evolve as the business and world, changes? When those answers are yes, AI moves from experimentation to effective execution.
Shaping AI from a smaller start
When it comes to effective execution, small models provide certain advantages. SLMs are compact, efficient alternatives to LLMs, designed to deliver strong performance for specific tasks while requiring significantly fewer computational resources. For instance, a 10-billion parameter SLM can be optimised for enterprise applications at a fraction of the cost and complexity of a much larger 400-billion parameter LLM. SLMs also maximise hardware use, reducing power consumption and heat generation, lowering operational costs
SLMs prioritise what truly matters for enterprises. They enable organisations to integrate AI with private, secured datasets, reducing exposure to third-party risks and ensuring compliance with industry regulations. Purpose-built for task and domain-specific applications, ranging from assisting with financial fraud detection systems to healthcare diagnostics, SLMs can be fine-tuned for greater precision while maintaining efficiency.
Their smaller footprint also makes them more flexible and easier to deploy in hybrid cloud environments, allowing businesses to run AI where it delivers the most value – whether on-premises, in private clouds or at the edge. Furthermore, adopting SLMs doesn’t require a massive technological overhaul.
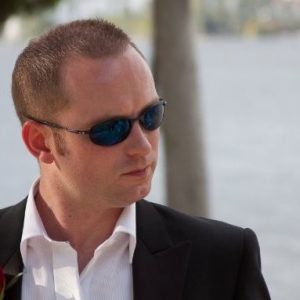
Red Hat’s Jerrom: Focus on right-sized AI to tailor solutions to specific business needs.
It’s important to commence rollouts by defining a model’s set purpose. This can help reveal where AI adoption makes sense and where it does not. Focused use-cases where SLMs excel include automating customer support, enhancing fraud detection and improving operational efficiency through fine-tuned insights. Once a model’s use case has been defined, SLMs like IBM Granite models can be adopted strategically.
Small & large models together
While SLMs are clearly becoming foundational, most enterprise AI strategies involve more than one type of model. Enterprises are adopting hybrid AI architectures that leverage the broad capabilities of LLMs for general-purpose tasks, while employing SLMs for domain-specific functions, thereby optimising performance and resource utilisation. When used together, they form a layered architecture that aligns with how modern enterprises already build and run software.
It’s not just that SLMs can complement LLMs – it’s that they unlock different strengths. SLMs can be deployed to handle specific tasks or domains, co-located with data sources, tuned to local compliance requirements and easily iterated on. LLMs, meanwhile, serve as general-purpose engines for inference, summarisation or decision support – running in a more centralised fashion where scale makes sense.
This layered approach reflects how I see the future of intelligent systems: distributed, interoperable and modular by default. In a hybrid environment, intelligent orchestration becomes key – routing queries to the right model, managing dependencies and ensuring that decisions are made in the right place, with the right context.
As this shift takes shape, the real opportunity in AI lies not in scaling up, but scaling smart. By focusing on right-sized AI, enterprises can tailor solutions to their specific business needs, ensuring compliance, cost-effectiveness and seamless integration into hybrid cloud environments.